Data-Driven Decision-Making in Healthcare: Paving the Way to Success
- Jessica Morley
- Nov 17, 2022
- 7 min read
The other day I gave a 15 minute overview of my DPhil thesis to Exeter College Oxford & it was so fun I thought I’d thread it here. SO I present: “Data-Driven Decision Making in Healthcare: Paving the Road to Success.” Or how to ensure the use of CDSS (especially algorithmic CDSS) helps more than it hurts.
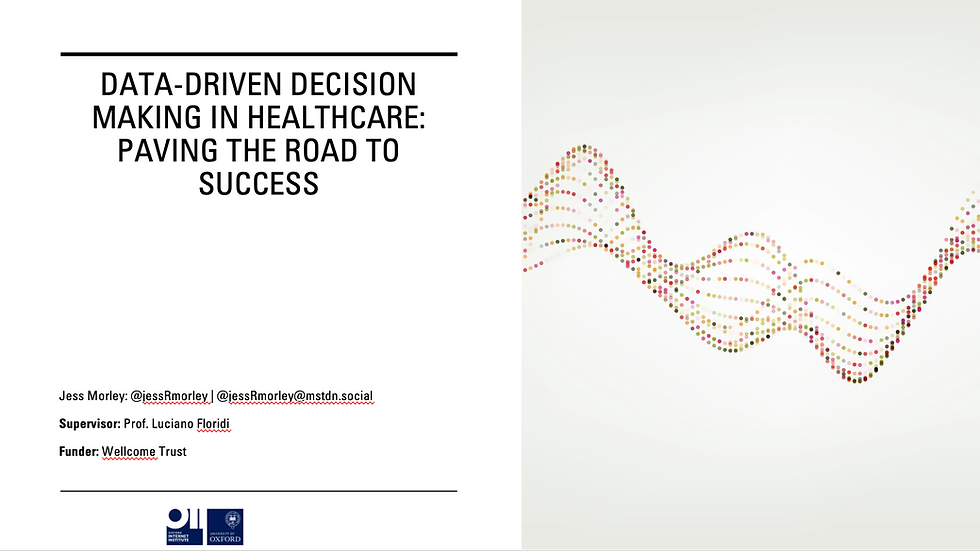
The NHS was founded in 1946 with the aims of meeting the needs of everyone and being free at the point of care. It has been a phenomenal success, but it is undeniable that it is now under considerable stress.
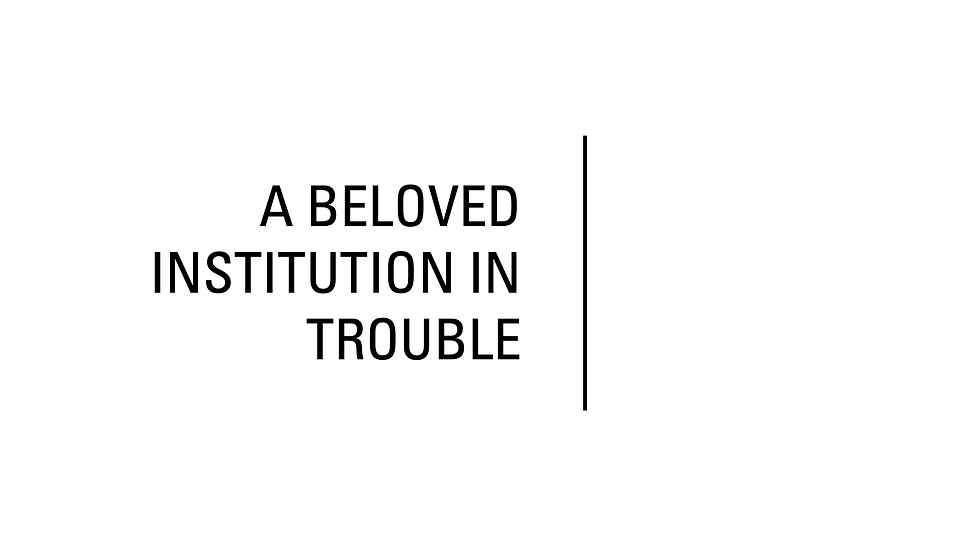
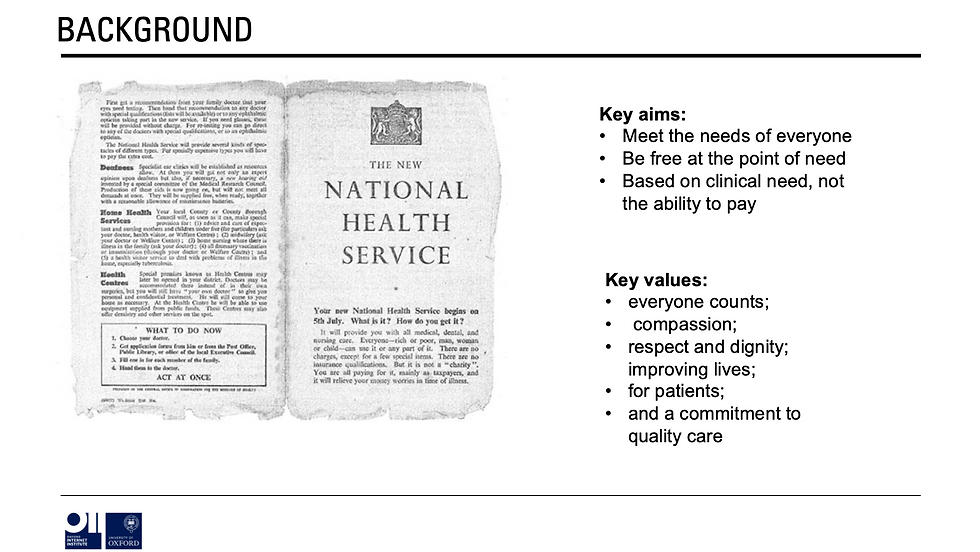
21st century patients are very different to 20th century patients. Life expectancy has increased, as has the number of people living with long-term conditions and dealing with multi-morbidities. The deficit was >£910million BEFORE COVID and waitlists are higher than ever.

Increasingly, policy and strategy documents present ‘P4 medicine’ as the ‘solution’ for helping the NHS achieve the ‘aim’: improved population health, improved experience of care, and reduced per capita cost.
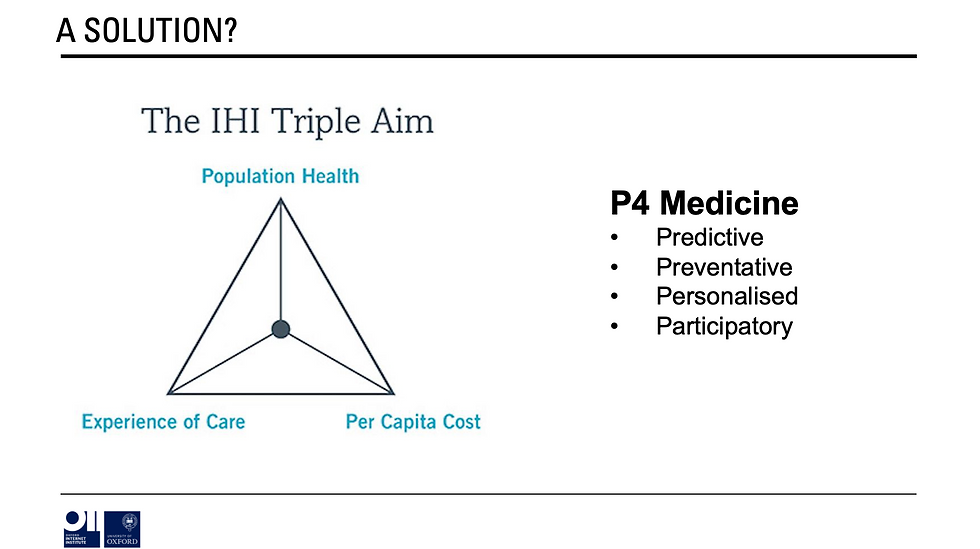
The hope is that if we can predict when a person (or groups of people) are going to become unwell, we can intervene earlier, target the treatment to them - based on richer information about their bodies and lives - then we can reduce the cost of care.
This idea rests heavily on the use of data. More data about the factors associated with ill health. More data about how different people react to different treatments. More data about trends in population health etc. etc. This, in itself, means more use of analytics (inc but not exclusively AI).
So you get policy documents infused with rhetoric such as:
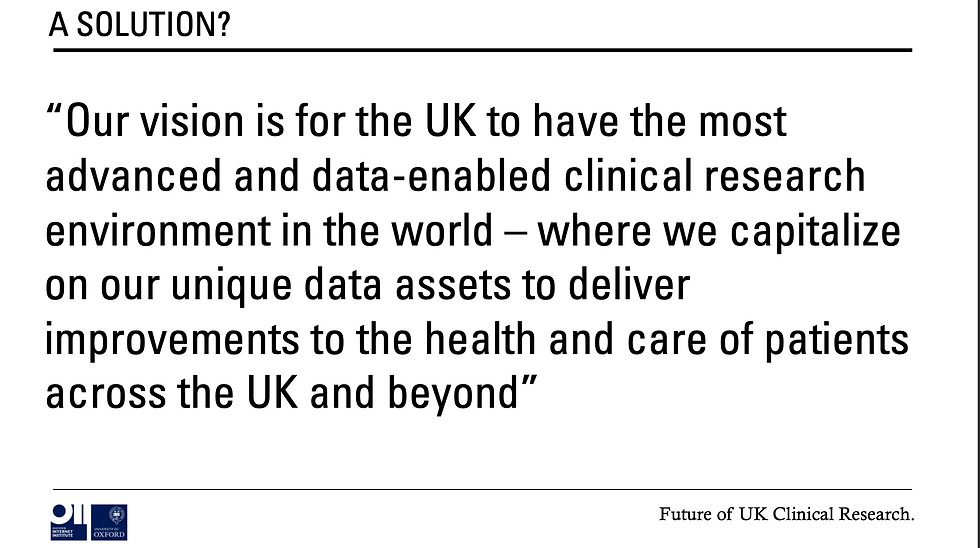

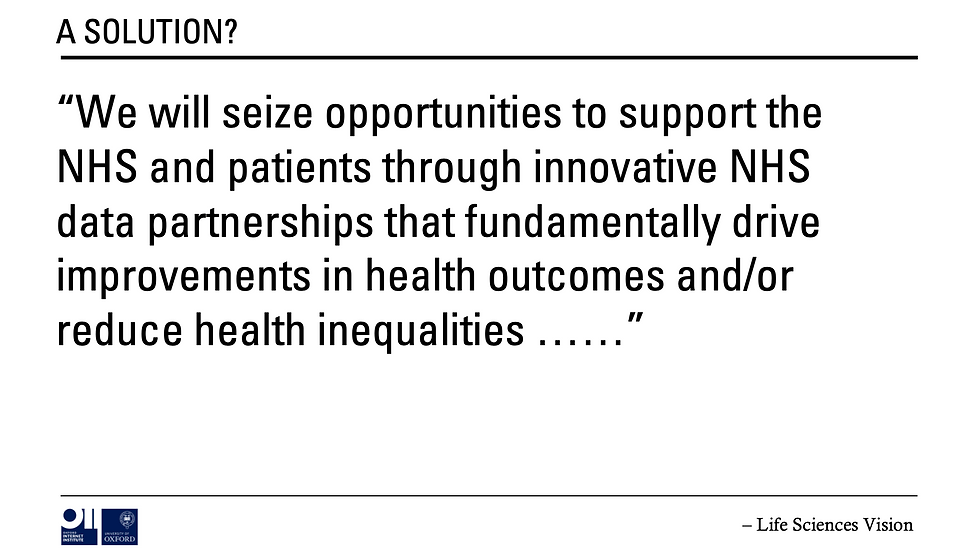
Implementing these ideas i.e. moving them out of policy rhetoric and theory into the ‘real world’ requires (even if it’s not always explicitly stated) the greater use of clinical decision support systems (CDSS) particularly algorithmic or automated CDSS (ACDSS).
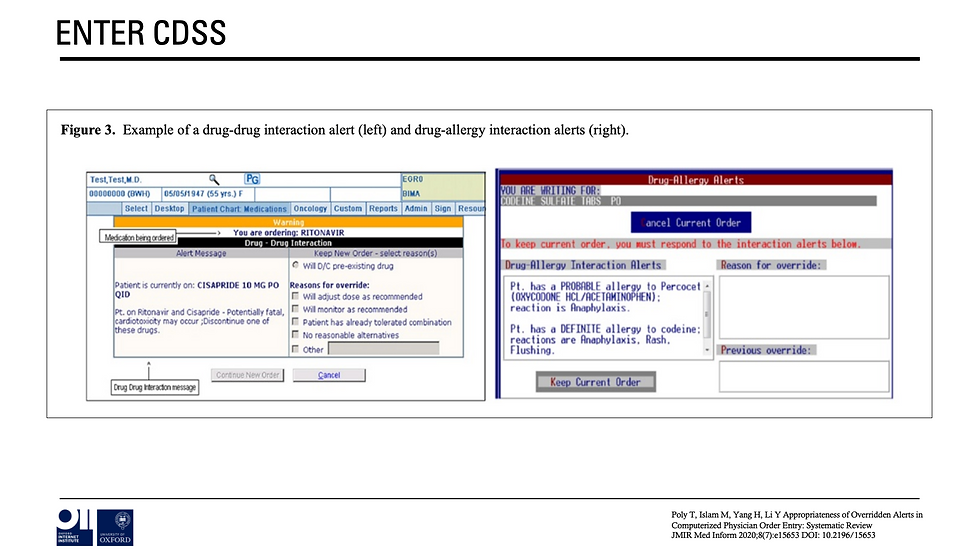
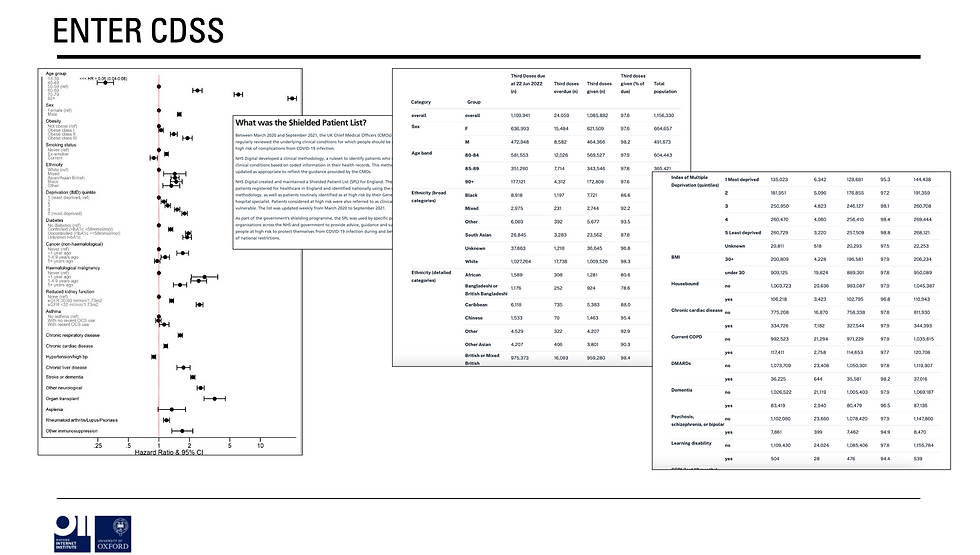
The idea being that if (for example) you can create complex models that identify factors associated with disease, these models can be deployed in clinical systems to highlight to clinicians which patients on their list should be invited for screening / intervention etc.
OR at the point of care a pop-up could tell a doctor what would be the most appropriate drug to prescribe for that particular patient based on a range of factors (recorded in a patient’s electronic health record) that (e.g.,) research identified as being influential in terms of reactions.
This all sounds great in theory. BUT the reality is more complicated.
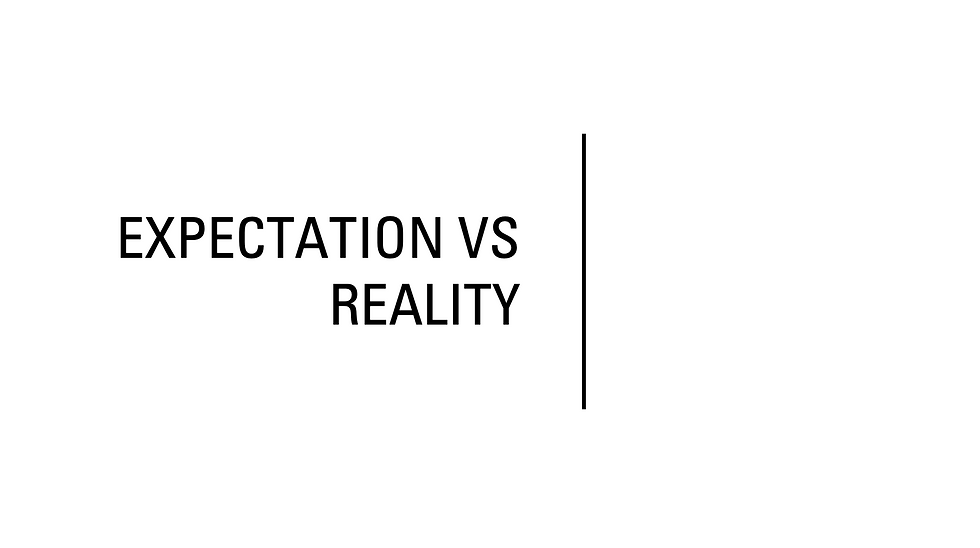
First, there is a BIG gap between what the system is currently used to and currently capable of delivering, and what is being proposed in these visions of a ‘data-enabled learning healthcare system.’
As Rob Wachter says of most applications of advanced analytics: “there is more promise than reality and more hype than evidence.” This slides shows that in the same month the NHS released its buyer’s guide to AI & lost 000s of COVID results by using the wrong excel format.
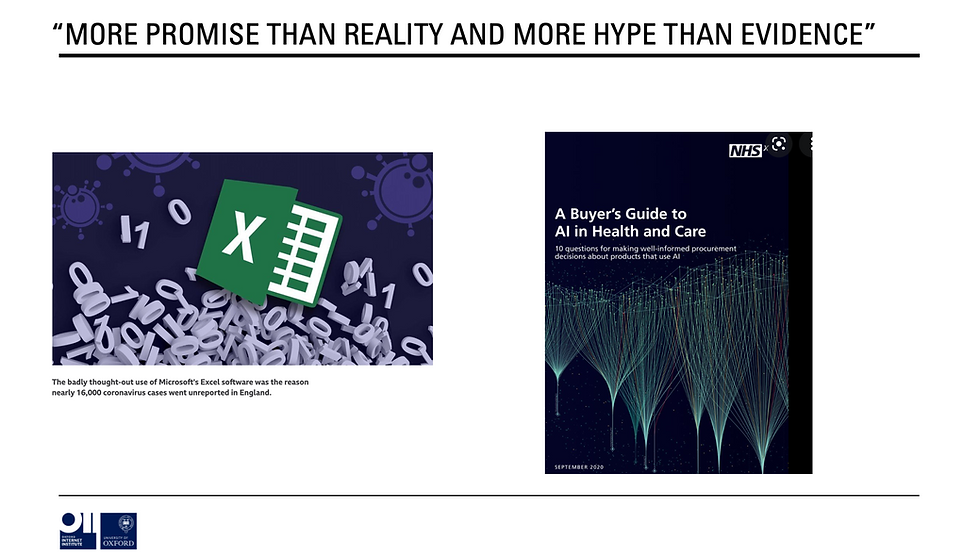
If this was an Instagram account the expectation vs. reality difference would be HUGE.
Second, the NHS has historically struggled with technology transformation at scale. Or, at least, the process is never as straightforward as initially expected. NPfIT was famously over budget and underwhelming in terms of delivery (although we did get the NHS Spine).
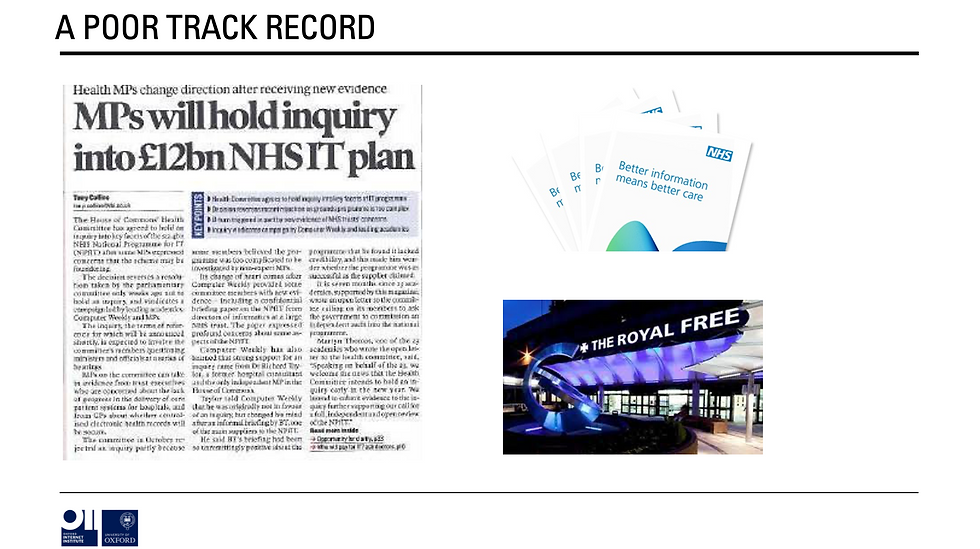
Similarly, projects such as care.data, DeepMInd and the Royal Free, and the more recent GPDPR programmes have run into trouble due to a combination of technical, regulatory, social, and ethical concerns.
So this raises the question. Should we just give up?

Well no, of course not. The NHS constitution commits to the use of any innovation that has the possibility of saving lives. This is in both its principles and values and is underpinned by a number of foundational human rights arguments.

We know that CDSS - which is categorically NOT a new idea- can save lives when thoroughly tested and implemented well. The trick is to be mindful of the benefits AND the risks as well as the appropriate constraints.
Which brings me to the motivation, aim and overarching research question of my thesis. Motivation: help the NHS capitalise on the opportunities of ACDSS whilst proactively mitigating the risks.
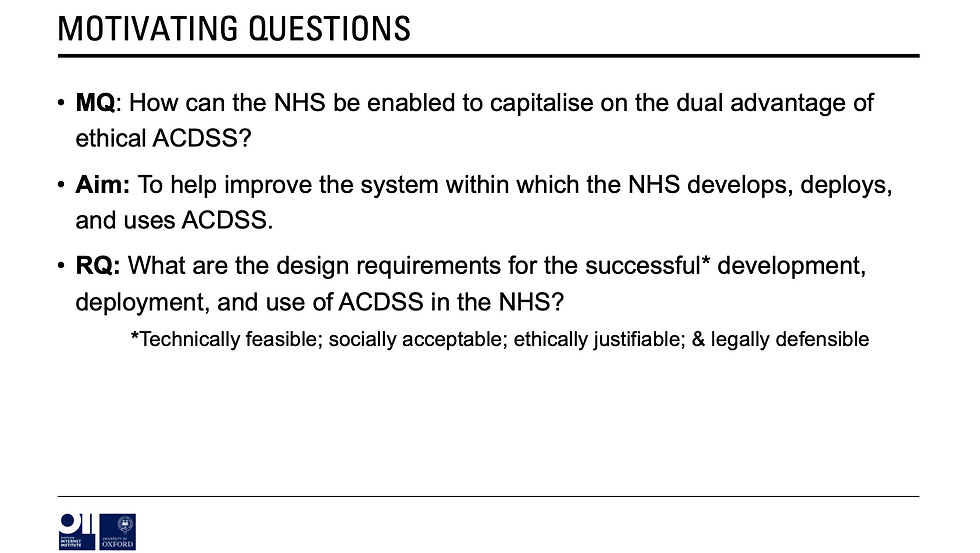
Aim: Improve the system within which the NHS develops, deploys, and uses ACDSS, by answering the research question: what are the design requirements for the successful development, deployment, and use of ACDSS in the NHS?
Success in this context is defined as technically feasible, socially acceptable, ethically justifiable and legally defensible.
To do this we must embrace the logic of design (H/T @floridi). By this I mean think about how we bring a system conducive to ‘success’ into reality, rather than throwing ACDSS into the current system on a wish and a prayer that it will work.
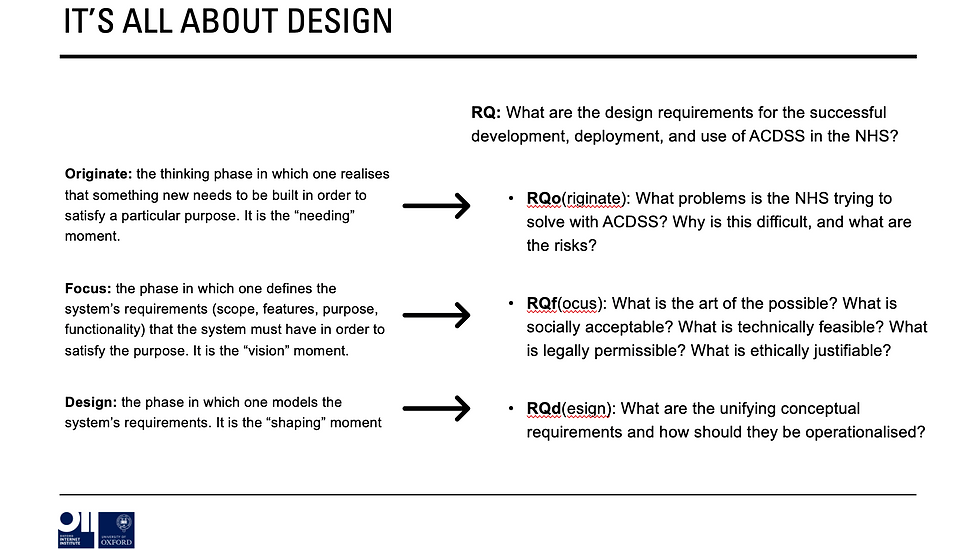
So, we must break the research question into three: originate (what hasn’t worked, what should we be trying to achieve, what are the risks?); focus: (what is the art of the possible?); and design (what are the requirements & how should they be operationalised?)
But this must have been done before!?!?! I hear you exclaim.
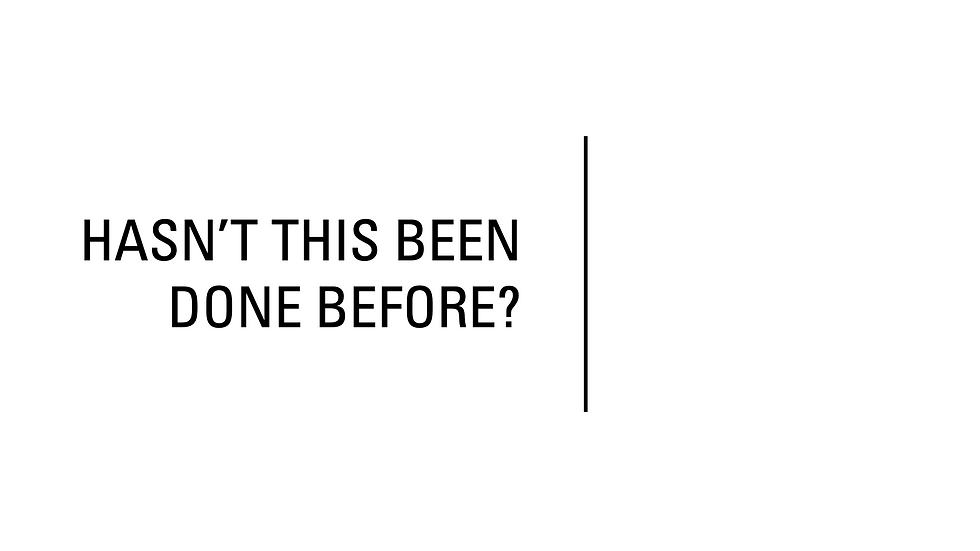
Well, kinda. There is literature about achieving change in the NHS; Adoption, diffusion & spread; methods for developing ACDSS; the ethics of AI; & the regulation of AI. But very often literature in these fields treat technical, ethical, social, and legal requirements as somehow siloed.

This is unlikely to result in success. Indeed, case studies of past failures show that one of the main underlying causes of failure is lack of understanding of complexity i.e., a lack of recognition of how factors in these areas intersect, interact, and result in emergent uncontrolled effects.
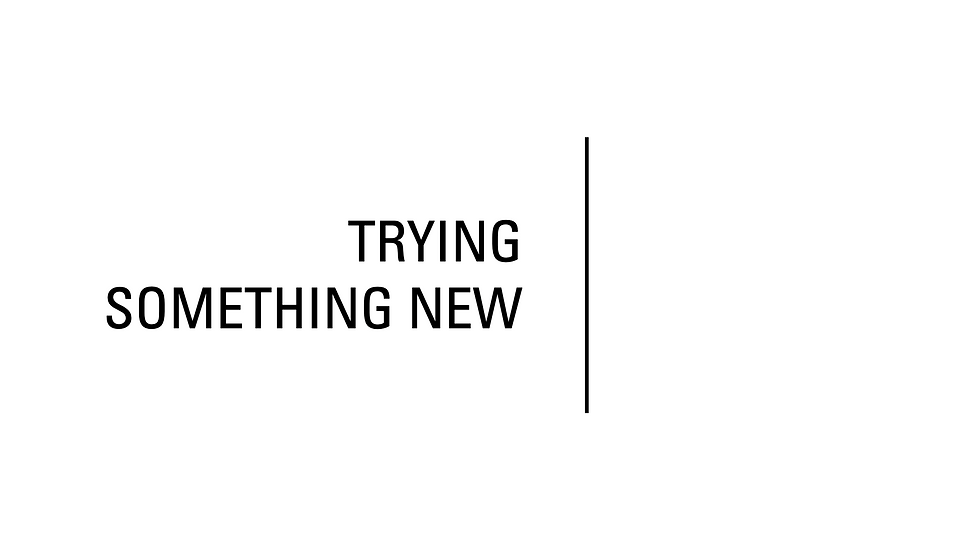
To increase the chance of ‘success’ therefore, we need to take a system-level approach informed by complexity and implementation science, one that sees technical, ethical, social, and legal requirements as inherently intertwined.
This is where I come in. My thesis attempts to do just this by combining ‘data’ (sorry quants) from a realist literature review; a policy analysis; and a series of semi-structured interviews, analysing these results and developing a theory-informed narrative synthesis.

(If you’re really into numbers, then it’s 180 academic papers, 42 policy/legal/strategy documents, and 75+ interviews with policymakers, academics, clinicians, technologists, and patients)
Let’s see what happens when you stitch it all together shall we?
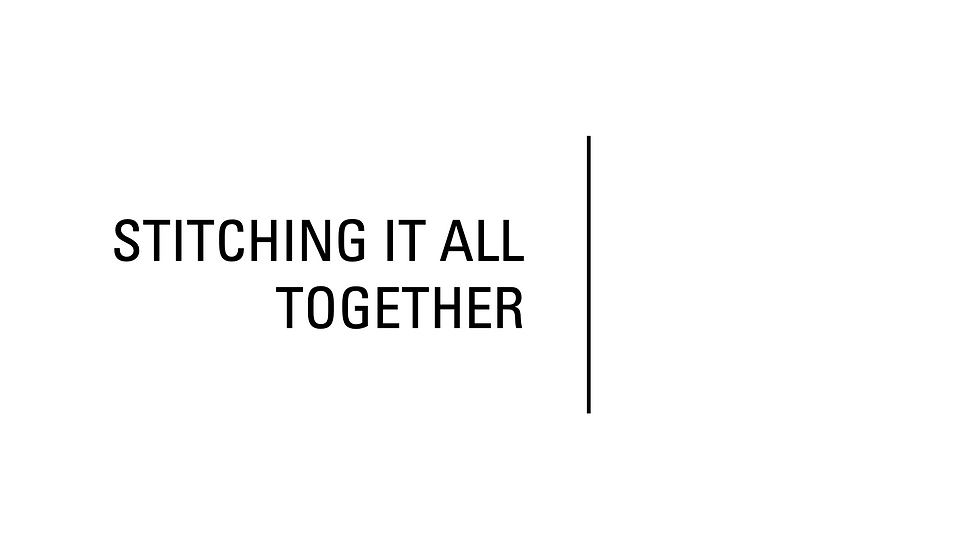
Well first of, it transpires, that designing, developing, implementing, and using ACDSS in the NHS is SUPER HARD. If this was 2008 and we all still updated our relationship statuses on Facebook then the relationship between the NHS & ACDSS would be “it’s complicated.”
If we look at the NAASSS Framework from @trishgreenhalgh et al. then we can see that the way in which ACDSS is currently positioned in policy rhetoric results in a ‘complex’ score in each of the highlighted 7 domains……
….. Condition; Technology; Value Proposition; Adopter; Organisation; Wider System; and Embedding Over Time. There is, therefore, a fairly urgent need to reduce complexity if success is to be even remotely possible.
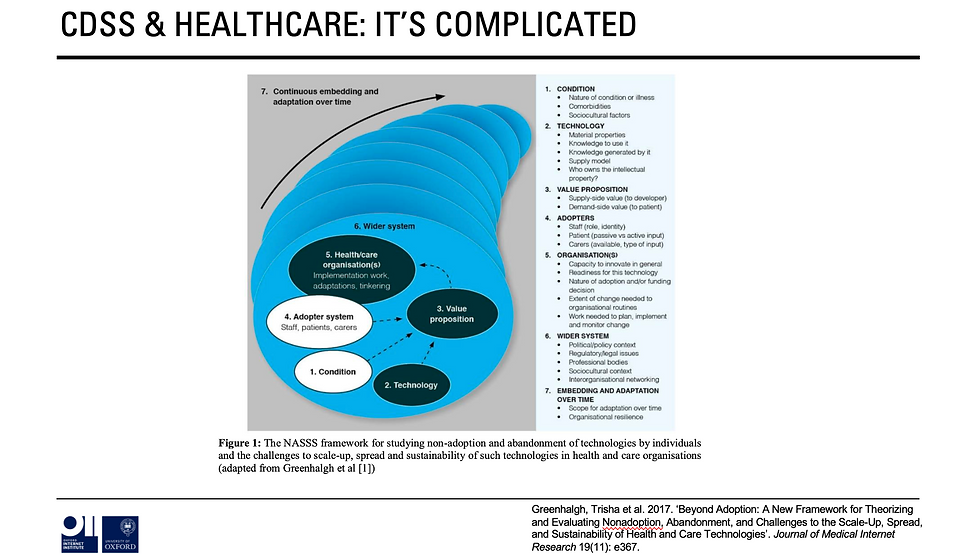
In addition, there are considerable social and ethical risks that need to be taken into account. Without going into detail, unless carefully designed the at-scale use of ACDSS could fundamentally alter:
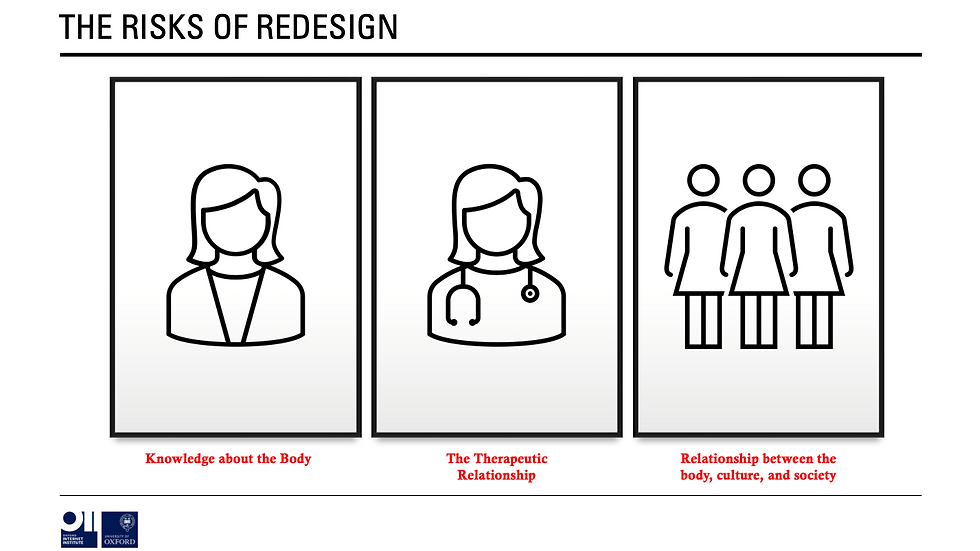
What counts as valid ‘knowledge about the body.’ ACDSS can easily take into account things that are measurable in a quantifiable sense e.g., weight, height, but not things that are more qualitative or subjective e.g., ‘how you feel’ or the social determinants of health.
The therapeutic relationship. Even if we don’t fully understand why, we know that core tenets of ‘patient-centric’ and ‘shared-decision-making’ are essential to good outcomes in healthcare. In others words empathy, autonomy etc. matter and it’s hard to design human+machine systems that protect these essential aspects of care.
Relationships between the body, culture, and society. Algorithms are quite stupid. They are massively impacted by data quality, and they also cannot account for context. This has implications for discrimination, ‘blame culture,’ and the position that ‘wellness’ etc. holds in society.
With these considerations in mind then we can move onto considering barriers and enablers of success using the ITPOSMO framework. It turns out there are many from the importance of epistemic certainty to the need for legal clarity and the importance of developing a workforce skilled in a diverse range of areas.
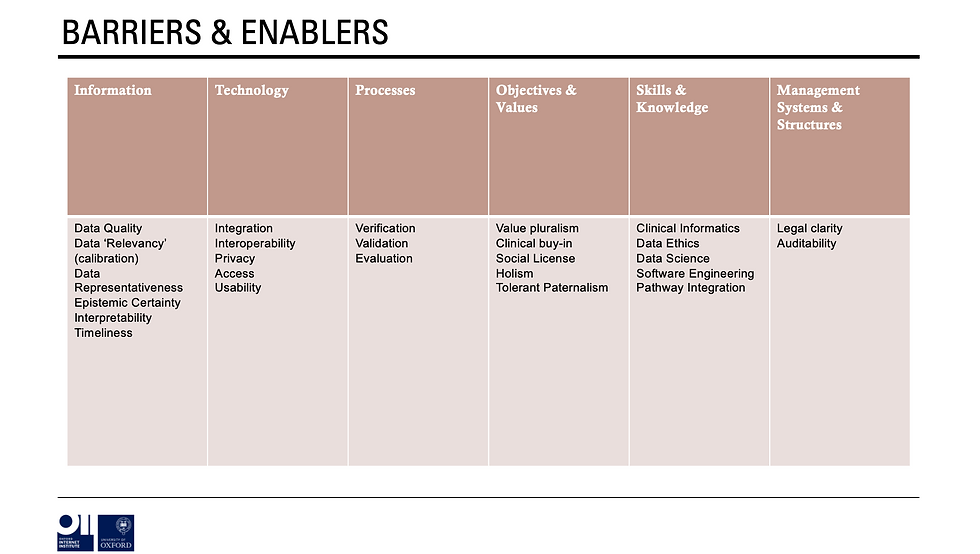
Once these barriers & enablers have been elucidated, then we can see how many are covered by current policies, strategies, legal documents etc.
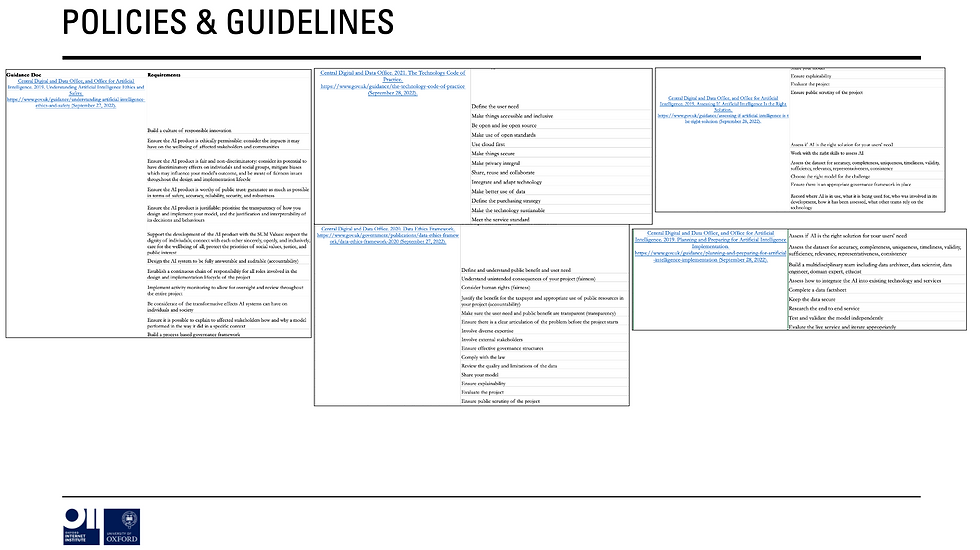
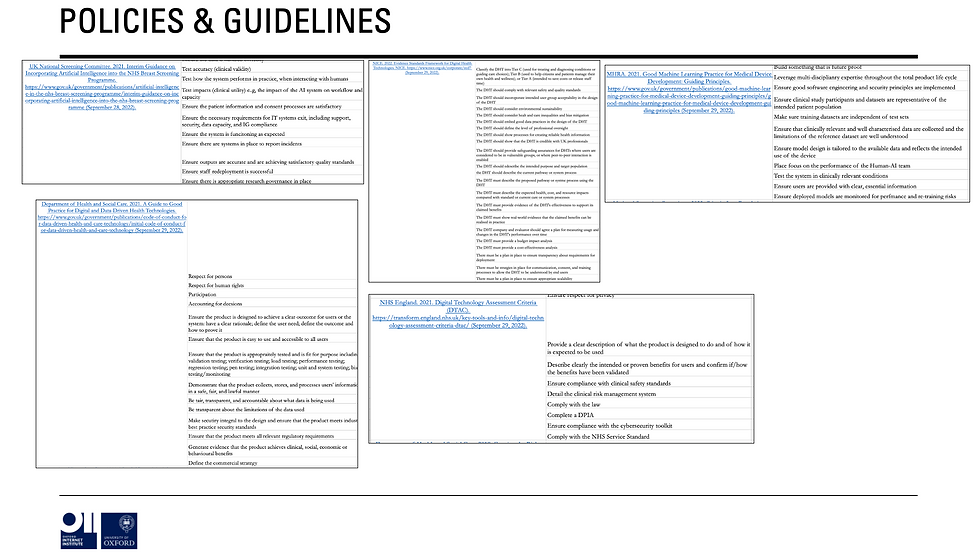
Here, it’s clear that many of the main barriers and enablers are referenced at a high-level in various documents BUT often in vague, unspecific ways that focus on the what and not the how (sorry I’m a broken record on this point).
In addition, to find how policy (broadly defined) is trying to tackle these barriers and enablers you have to look ALL OVER THE PLACE from medical device law, to good design guidelines from the Office of AI, to the principles underpinning decisions made by the National Screening Committee.
And, shock, none of them reference each other nor consider how they sometimes contradict each other etc. Plus virtually none take into account the importance of ‘culture’ or the social factors that are equally as important as technical/legal.
What’s more, policy and strategy documents frequently make a number of assumptions that are never made explicit. And what this makes clear is that the ‘theories’ policy/strategy are using to guide their design of the ‘system’ are very different to those that would be used by others (inc. academics, clinicians, and patients).
In a nutshell it’s clear that much of policy rhetoric (including rhetoric in some parts of the academic literature) is heavily infused with a deterministic attitude that would benefit from a reminder that……
….”there is no single development, in either technology or management technique, which by itself promises even one order-of-magnitude improvement within a decade in productivity, in reliability, in simplicity.”
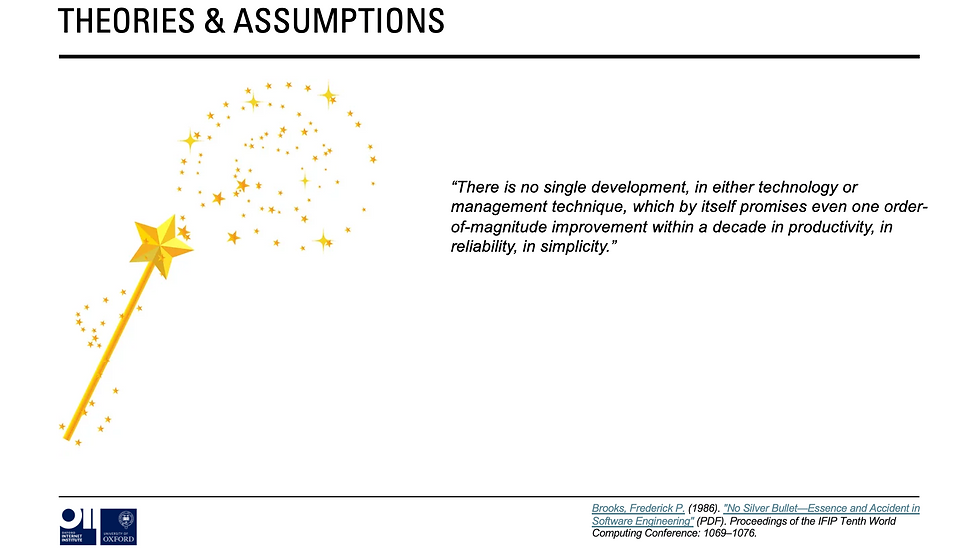
To overcome these hurdles then, we must rethink how to design the system based on a series of unifying concepts: utility, usability, efficacy, and trust and then consider how to operationalise these.

And for now, this is where I will stop. Much more to come! Thanks for listening.
Discover Your Exact Age Instantly with Our Age Calculator! Ever wondered exactly how old you are—not just in years, but down to the months, days, hours, or even minutes? Whether you're planning a birthday, completing official paperwork, or just curious, our Age Calculator gives you the answer in seconds!
🧠 What Can It Do?
Our powerful tool isn’t just a basic birthday counter. With just one click, it calculates your precise age from your date of birth, showing:
✅ Years, months, days, hours, and even minutes
✅ The day of the week you were born
✅ Fun facts like zodiac sign and age milestones
✨ Why Use Our Online Age Calculator?
Perfect for students working on projects
A helpful tool for HR professionals and…